Good Character AI Alternatives: Exploring Effective Alternatives to Traditional AI
In the realm of artificial intelligence, the search for ethical and character-driven alternatives to traditional technologies has gained momentum. As we strive for more humane and ethical AI solutions, it is essential to explore alternative approaches that prioritize not only efficiency but also values like transparency, accountability, and fairness. In this blog post, we delve into the concept of good character AI, examining its significance and exploring alternative approaches that offer a more ethical and responsible framework.
Understanding Good Character AI
Good Character AI goes beyond mere functionality; it embodies the principles of ethical design, human-centric values, and responsible decision-making. Unlike traditional AI systems that operate based on predefined algorithms and data sets, good character AI emphasizes traits like empathy, transparency, and inclusivity.
Key Attributes of Good Character AI
- Empathy: Understanding and responding to human emotions and needs.
- Transparency: Openness in decision-making processes and outcomes.
- Fairness: Ensuring unbiased and equitable treatment for all individuals.
Exploring Effective Alternatives
Section 1: Human-in-the-Loop AI
Human-in-the-Loop AI combines the strengths of AI algorithms with human oversight and intervention. By involving humans in the decision-making process, this approach enhances accuracy, reduces biases, and ensures ethical considerations are prioritized.
The Role of Humans in AI Decision-making
Individuals play a crucial role in supervising AI systems, providing insights, and correcting errors to maintain ethical standards.
Section 2: Federated Learning
Federated Learning enables collaborative AI model training without compromising data privacy. By distributing the learning process across devices or servers, this approach safeguards sensitive information while improving AI capabilities.
Ensuring Data Privacy in Collaborative AI
Protecting user data and privacy remains a primary concern in the development of AI technologies. Federated Learning offers a solution that addresses these concerns effectively.
Section 3: Explainable AI
Explainable AI focuses on transparency and interpretability, allowing users to understand how AI systems reach specific decisions. By providing clear explanations and justifications, this approach enhances trust and accountability.
Enhancing Trust through Transparency
Enhanced transparency fosters trust between users and AI technologies, enabling informed decision-making and ethical practices.
Key Takeaways
As the demand for ethical and responsible AI continues to grow, exploring good character AI alternatives is essential. Incorporating concepts like empathy, transparency, and fairness into AI design can lead to more ethical and trustworthy systems. By embracing approaches such as Human-in-the-Loop AI, Federated Learning, and Explainable AI, we pave the way for a more inclusive and humane AI landscape.
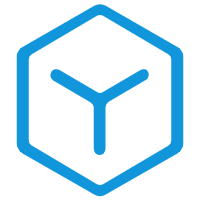
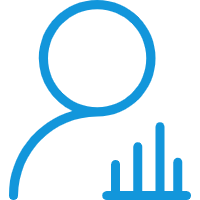